Neural Field Convolutions by Repeated Differentiation
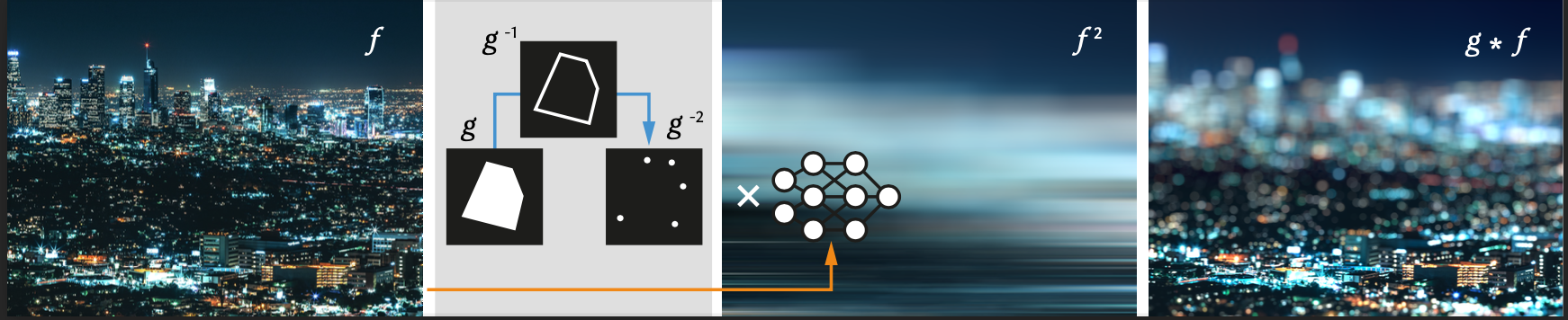
Abstract
Neural fields are evolving towards a general-purpose continuous represen- tation for visual computing. Yet, despite their numerous appealing proper- ties, they are hardly amenable to signal processing. As a remedy, we present a method to perform general continuous convolutions with general continuous signals such as neural fields. Observing that piecewise polynomial kernels reduce to a sparse set of Dirac deltas after repeated differentiation, we leverage convolution identities and train a repeated integral field to efficiently execute large-scale convolutions. We demonstrate our approach on a variety of data modalities and spatially-varying kernels.